In our last post, we looked at the theory that 'confidence' was related to uncertainty about a 'true' probability. This was the first of seven theories that have been put forward as to how confidence judgements should be interpreted. In this post, we'll look at the next two theories - 'Information' and 'Ignorance'.
These two theories are closely related, and suggest respectively that 'confidence' is related to the amount of information that has been used to form a judgement, or that it is related (inversely) to the amount of information missing from a judgement.
The 'Information' theory suggests that the difference between
"I think there's a 70% chance of rain, and I am very confident in that judgement"
and
"I think there's a 70% chance of rain, but am not very confident in that judgement"
should be interpreted as something like the difference between
"I have a lot of information suggesting there's a 70% chance of rain"
and
"I think there's a 70% chance of rain but I don't have much information to support that"
Photo: Biswarup Ganguly
The 'Ignorance' theory, meanwhile, would parse them as something like the following:
"I've seen nearly all the available information, and it suggests there's a 70% chance of rain"
and
"I think there's a 70% chance of rain, but there's a lot more information I could get hold of that might give me a better idea".
As with all the theories of 'confidence', we'll put the 'Information' and 'Ignorance' ideas to three tests: internal coherence, usage by analysts, and decision-relevance.
Are the theories internally coherent?
Both the 'Information' and 'Ignorance' theories depend on a crucial claim: that information 'quantity' (either available, or missing) can be meaningfully separated from the probability judgements that the information helps support. This claim seems superficially plausible. These two statements do seem different in a relevant way:
1. Based on an article I read in a magazine, I'd say there's a 20% chance that William Shakespeare wasn't the author of the plays normally attributed to him.
2. I've read everything that's been published about Shakespeare, and I think there's a 20% chance that he wasn't the author of the plays normally attributed to him.
Let's assume our analysis is well-calibrated (that is to say, out of every five statements we attribute a 20% probability to, one turns out to be true): is there a relevant difference between these two statements, in terms of any force with which I should act on the claim? It certainly feels like the latter claim is better supported - that it has more information in it, or perhaps lacks less information - but can we pin this feeling down to something measurable?
In the previous post, we touched on the mathematical field of information theory in the context of the claim that 'probability' does not refer to a measurable feature of systems, as such, but that it quantifies information, or, equivalently, uncertainty, about those systems. This view of information, first described by Claude Shannon in 1948, implies something very important: that you cannot meaningfully talk about a 'low information' 20% and a 'high information' 20%, any more than you can talk about someone being a 'short' 1.7m in height as opposed to a 'tall' 1.7m, because the information content of a judgement is encapsulated by the probability.
But might there be other ways of defining 'information' that don't entwine it so closely with probability, and which would accord with what the 'Information' and 'Ignorance' theories of confidence purport to describe? Possibly. Based on conversations with them, and statements from survey participants, it seems that some analysts have a model of 'available information' that treats it like a finite 'in-tray' of possibly-useful material, or 'raw data', which is then 'ingested' as evidence. In the 'in-tray' model, 'information' is measured not in terms of its impact on the probability of hypotheses of interest, but in terms of something like 'bytes ingested' versus 'bytes remaining'.
The in-tray model does make an interesting and possibly-useful distinction between probability, information used, and information remaining. It allows for 'useless information' that does nothing to change a probability. It also makes the distinction between information and ignorance meaningful: 'information' is how much of the in-tray you've ingested, while 'ignorance' is how much is left; it's possible to have low information and low ignorance, or high information and high ignorance - a situation that the Shannon measure doesn't admit.
We can loosely illustrate the differences between these interpretations as follows. Suppose there are three papers that might shed light on a hypothesis of interest - say, the efficacy of a certain drug in treating hayfever. The first, short, paper details a preliminary study that provides some evidence of effectiveness. The second, longer, paper outlines a large-scale clinical trial that provides strong evidence in its favour. The third, also lengthy, paper turns out to be of no relevance. The difference between the 'in-tray' and the Shannon interpretations of 'information' might look a bit like this:
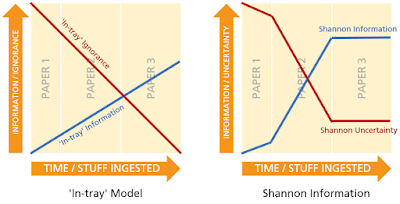
The problem with the in-tray model is that the concepts it relies on are vulnerable to fairly-arbitrary changes in conditions. Inserting irrelevant data into the 'available data' bucket will raise one's ignorance without affecting one's judgements, while reading it will then add to 'information content' without affecting their evidential basis. We might be tempted to respond by changing our definition from 'information' to 'useful information' - but then we are back to square one, for how else can we define 'useful' information other than in reference to its effect on the probability of the things we are interested in?
In summary, the 'information' and 'ignorance' theories of confidence can only be made coherent if they rest on a concept of 'information content' that differs from the orthodox information-theoretical measure, which treats probability as a measure of information. But measures of information which divorce information from probability are vulnerable to seemingly-arbitrary distortion by 'useless' information.
Does the theory align with usage?
There is evidence that many analysts think of 'confidence' in terms of 'how much information' underlies a judgement. The responses to our survey, which invited analysts to define 'confidence', included the following:
"Confidence here means the extent to which, given your knowledge of the data you do have and (importantly) the known gaps, you assess that your statement/analysis is likely to be accurate."
"Confidence here means how well the probabilities are based on reliable statistics and good data samples. (Many of the supplementary bits of information are irrelevant because they do not change the available information.)"
"Strength of an opinion based on all available evidence, factoring in unknowns."
(Although to the extent that the survey responses could be clearly classified, this was an interpretation offered only by a small minority of respondents.) One thing however is clear: that whatever analysts use 'confidence' to mean, they do not on average have in mind something related to the Shannon definition of 'information content'. The following chart shows the relationship between the information content implicit in the probabilities presented, and the mean subjective confidence scores survey respondents assigned to them.
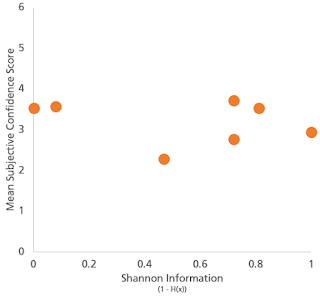
If respondents' confidence scores were in some way capturing Shannon information, there would be a strong positive relationship between these two - and there isn't.
One of the survey questions was designed to test whether analysts thought of information in 'in-tray' terms. In it, analysts were presented with a set of situations which involved reading a number of papers about the effectiveness of a travel sickness drug. The scenarios varied by the number of papers that had been read, and the number of papers that had not been read. Crucially, in every case the relevant information (i.e. Shannon information) was the same - that 1000 people (in total, across all the papers that had been read) had been tested, and the drug was effective in 750 of those cases. The table below shows the mean subjective confidence scores (from 0-6) that were assigned by survey respondents in each of the high / low 'information' and 'ignorance' cases:
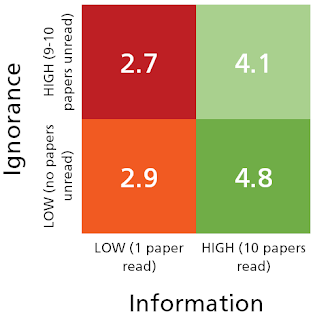
What these scores suggest is that analysts' confidence is indeed affected not by information in the Shannon sense, but by information in the 'in-tray' sense - how much 'stuff' they had read, and how much 'stuff' they hadn't. But the scores indicate that 'information' was more important a driver than 'ignorance'; reading 10 papers rather than 1 - even if the total data inside them were the same - raised scores significantly, but leaving papers unread had only a small effect.
These data may suggest that analysts are using an in-tray type model of 'information' - that if an analyst has read more things directed at a hypothesis, even if they are of limited relevance, their confidence goes up. This might indicate that analysts' perception of confidence is in some way related to their sense of having done 'due diligence' on an analytical problem - i.e. to their feeling of having 'done their job' - rather than to the amount of analytically-relevant information they've actually unearthed. There may, of course, be other explanations not tested by our survey.
Is it decision relevant?
Finally, if the 'information' and 'ignorance' theories of confidence were meaningful, would they be decision-relevant? Could knowing the confidence attached to a probability affect the optimal decision being made?
The answer is a qualified 'yes'. If 'confidence' communicates information content, and if that information content is not captured by the probability, then it might suggest to us whether we should act now (if there was already 'enough' information in the judgement) or to collect and analyse more information (if there wasn't). On the other hand, merely knowing how much 'information' went into a probability - if this makes sense as an idea - wouldn't by itself tell you how valuable new information is likely to be nor how much it would be expected to cost, both of which are key to deciding whether the next bit of information is worth investing in. We will look at these more-complex economic concepts later.
In the next post we'll examine the fourth theory of 'confidence' - the 'quality' theory, which posits that confidence is not reducible to a simple measure and instead captures summary judgements about the qualitative features of the evidence-base.